by Boaz Mizrachi, Co Founder and CTO, Tactile Mobility
When I talk about AI in vehicles, people might assume that I’m discussing autonomous vehicles or self-driving cars. While that’s certainly one facet of AI, and a fascinating one, there are many other applications for the technology long before the rubber hits the road.
In fact, the automotive industry is currently poised to rely more heavily on AI during vehicle design, production, and operation in the coming years. That’s likely why the global market for AI in automotive production is predicted to have a compound annual growth rate (CAGR) of nearly 40 percent between now and 2027.
It’s clear AI is already transforming how we both design and interact with our vehicles, and there are many more opportunities for the future. Companies just need to get a little more imaginative.
Design and development
AI is changing how vehicles are designed, developed, and tested. In other words, AI is changing not only the cars on the roads but the factories that produce them. These factories are deploying AI-powered robots, collecting AI-enabled data, and using the results to make data-driven design decisions.
AI algorithms can analyze vast amounts of data, including customer preferences, market trends, and performance requirements, to assist designers in creating optimal vehicle designs. Moreover, by considering factors such as aerodynamics, weight distribution, and structural integrity, AI can suggest design modifications to improve efficiency and safety.
The tech also allows for more efficient testing and trials with what’s called a digital twin, a virtual model of a physical asset. With a digital twin, engineers can test every vehicle component to ensure it’s safe and effective before creating a physical prototype and wasting time and resources on a nonfunctional or flawed result.
Intelligent connectivity
By 2030, 95 percent of new cars will be connected to vehicles that can send and receive real-time data from onboard computers and sensors. These connected cars – which have been called “computers on wheels” – will be increasingly powered by AI that can automatically upload data into a shared platform or cloud.
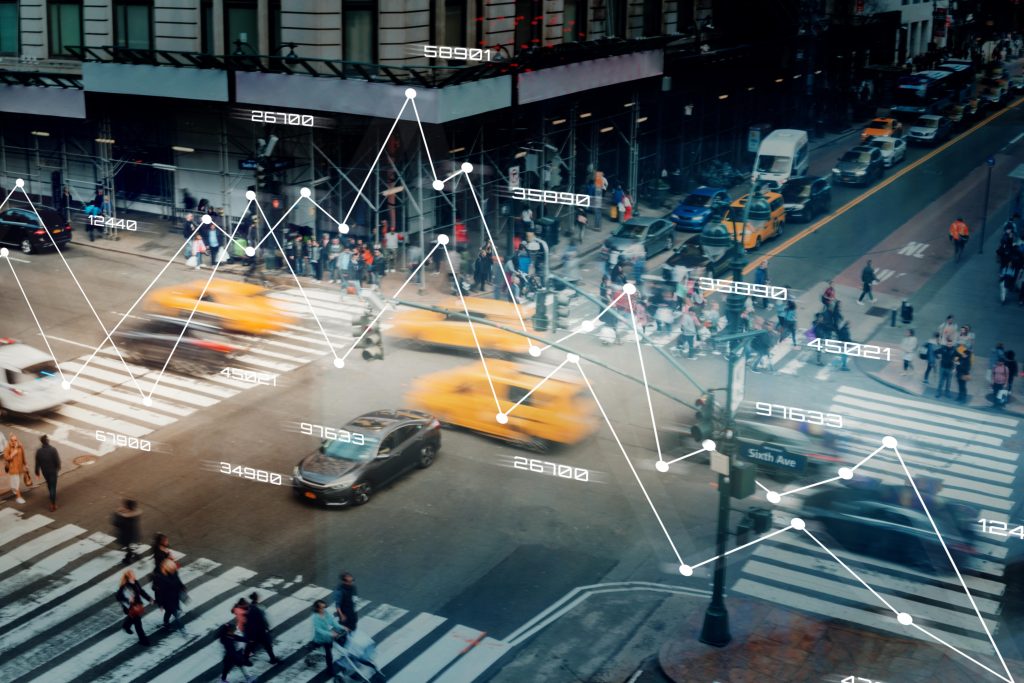
This level of connectivity will allow vehicles to communicate with each other and the cloud. That allows data to be collected, analysed and interpreted remotely minute by minute. This has significant implications for fleet operators, who can put hundreds of vehicles in a fleet online in the same system to ease management and deployment. This process is already happening in fleets from Amazon to Uber.
Moreover, all this data generated in real-time can make our roads safer. Cloud-connected vehicles with AI-powered sensors can capture data about road conditions – including potholes – and automatically report problems to local municipalities for repair.
Predictive maintenance
Think of a robot who lives in your car, whose entire job is to monitor its health and performance. That’s another way AI is being used by manufacturers in vehicles: to understand how the vehicle actually behaves on the road and sense when something needs to be changed.
AI-powered systems continuously monitor the condition of vehicle components, such as engines, brakes, and suspension systems, and how they work together. The smart software can track variables like temperature, vibration, and fluid levels to identify deviations from normal operating conditions, which may indicate a potential failure. This level of predictive maintenance also minimizes repair costs because drivers can respond to the problem before it spirals into other forms of equipment failure.
Many people know that when fuel consumption increases, it normally indicates inefficiency changes in the powertrain and driveline. Yet, it might be due to other factors that are not implying efficiency degradation (e.g., increase in vehicle weight, ambient temperature, tire pressure, etc.). Therefore, we must consider several parameters to convert a “hunch” into a valid prediction. If accuracy and resolution of the prediction are important, then many more parameters need to be considered to compensate for 2nd and 3rd-order physical phenomena. This is so tricky to do so that conventional algorithms are not enough (normally, due to a lack of parameters and changing coefficients). Here comes the AI concept to help: solve those missing coefficient equations to produce high-resolution estimation at high-resolution accuracy.
Armed with historical data and patterns, AI algorithms can forecast maintenance requirements for specific components or systems. For example, they can estimate the remaining lifespan of a battery or predict when an oil change is needed based on past vehicle history.
Driver assistance
AI is also being used to assist drivers themselves, with AI having differing degrees of autonomy and power in decision-making. This comes primarily in the form of Advanced Driver Assistance Systems (ADAS), which use a litany of physical and virtual sensors to monitor the surrounding environment. ADAS can detect pedestrians, hazards, or obstructions and alert the driver or issue a response. Think of automatic braking when your car gets too close to the rear of another vehicle.
The underlying principle of ADAS is speed, to quickly capture and process information to provoke a response with less than a few seconds’ notice. That can only be done with smart systems capable of instant processing such large swaths of information. In other words, there’s no optimal ADAS without AI.
Final thoughts
Overall, AI is collecting, collating, and responding to data, which has implications for various stakeholders, from Tier-1 manufacturers to road authorities to drivers. It’s time we recognize the power of these applications. Autonomy may get the headlines, but there’s much more to discuss.
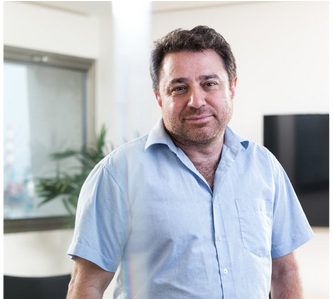
Author
Boaz Mizrachi, Co Founder and CTO, Tactile Mobility
Boaz is a veteran technologist and entrepreneur, holding over three decades of experience in signal processing, algorithm research, and system design in the automotive and networking industries. He also brings hands-on leadership skills as the co-founder and Director of Engineering at Charlotte’s Web Networks, a world-leading developer and marketer of high-speed networking equipment (acquired by MRV Communications), and as System Design Group Manager at Zoran Microelectronics (acquired by CSR).
Originally published on ManufacturingToday